AI’s Impact on Actuarial Methods and Its Transformative Potential
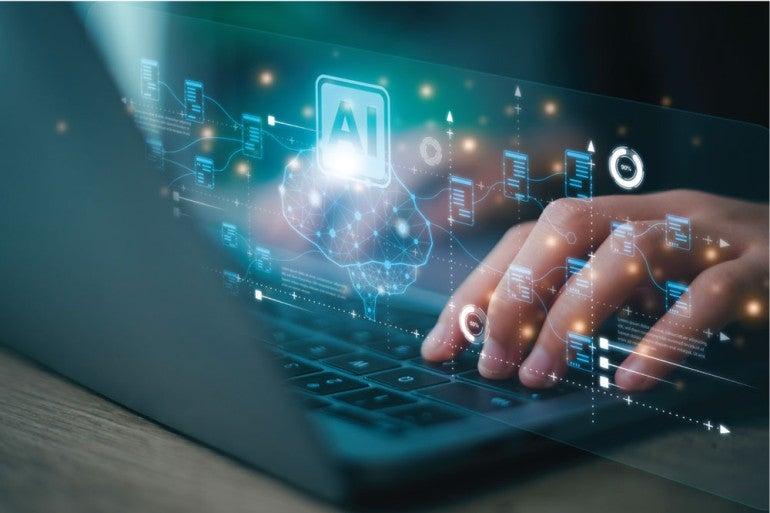
Milton Friedman, a distinguished economist and statistician, who was honored with the Nobel Memorial Prize in Economic Sciences in 1976, once famously said, “They [actuarial exams] are about the hardest examinations in the world, as anybody will tell you, in mathematics. I failed some and passed some.” Indeed, actuarial science has long been associated with complexity and rigorous mathematical analysis. Actuaries play a vital role in the insurance industry, estimating risks and managing financial outcomes, earning admiration and recognition from many stakeholders. Corporate entities like Uber, Lyft, Hertz, Google, Capital One and Expedia increasingly seek CAS members for their adeptness in technical training, business acumen and expertise in risk management (CAS, “Tesla CEO Touts Need For “Revolutionary Actuaries”). Notably, in 2020, the CEO of Tesla conveyed his high regard for the actuarial profession, emphasizing their mathematical prowess and expressing a desire for innovative actuaries. The potential of artificial intelligence (AI) to revolutionize conventional actuarial techniques stems from its capacity to harness extensive and detailed datasets. Traditional actuarial methods have served the industry well for decades, and AI appears to be a natural next step, offering innovative ways to enhance actuarial practices and improve the precision of risk assessment.
Len Llaguno, FCAS, of KYROS Insights, serves as an excellent example of this. He has shared how traditional actuarial practices, rooted in methodologies established decades ago, have primarily relied on overarching performance metrics and that these approaches frequently face challenges in adapting to the increasing complexities of insurance data and the surging need for detailed insights. To illustrate, the traditional reserving process primarily deals with aggregated claim data through well-established methods such as the chain ladder technique. He makes a noteworthy observation that, despite the availability of extensive data related to individual claims, the chain ladder often remains underutilized in the systematic estimation of ultimate losses and highlights how leveraging machine learning techniques presents as a readily available means to unlock untapped advantages of this data, potentially resulting in more accurate reserve estimates.
Notably, neural networks, which draw inspiration from the structural design of the human brain, play a pivotal role in this transformation. While they may appear as a novel concept, neural networks share fundamental mathematical principles with generalized linear models. Neural networks find applications in tasks such as claims forecasting, where they scrutinize historical claims data to unveil trends and forecast future claims volumes. Moreover, they empower insurers to gain deeper insights into customer behavior, facilitating the development of personalized insurance products and pricing strategies.
The influx of granular data not only enriches data analysis but extends to the pricing of insurance products. Initiatives like the CAS Hacktuary Challenge have spotlighted the inventiveness of actuarial professionals in crafting innovative risk engineering solutions tailored to the evolving landscape. For instance, “Risky Router” by Caesar Balona, a winning entry in the challenge, enables users to input their starting and destination points to obtain a dynamic risk assessment of their chosen driving route. Real-time factors such as weather conditions, time of day, vehicle age and driver impairment status are considered to provide a comprehensive risk estimate. Another innovative entry, “Consumer Vehicle Toolkit” by Michaël Bordeleau-Tassile, aids users in comparing insurance costs for different cars based on various factors like make, model and color. It also offers insights into areas with the highest likelihood of vehicle collision and theft in Toronto and Montréal, factoring in variables such as the time of year, time of day and road conditions (CAS, “CAS Announces Winners of $15,000 Hacktuary Challenge”).
The impact of AI on actuarial science extends beyond data analysis; it encompasses predictive modeling. AI-driven actuarial methodologies deliver real-time answers and insights. This dynamic adaptability empowers insurers to make more informed decisions, a crucial aspect in an industry where agility is of paramount importance. The amalgamation of AI and actuarial techniques signifies a substantial leap forward, enhancing the industry’s risk management capabilities and adaptability in an ever-evolving landscape, ultimately leading to improved financial outcomes. Overall, the integration of AI into actuarial methodologies represents more than a technological upgrade; it signifies a paradigm shift. Embracing AI as a potent tool in actuarial science promises greater accuracy, data-driven insights and a more agile response to the challenges of the modern world. As the insurance industry continues to evolve, AI stands ready to complement and elevate actuarial practices, ushering in a new era of risk assessment and management.